Chatbots and assistant programs designed to support conversations with human users rely on natural language processing (NLP). This is a field of scientific research that aims at making computers understand the meaning of sentences in natural language. The algorithms developed by NLP researchers helped power first generation of virtual assistants such as Siri or Cortana. Now the same algorithms are made available to the developer community to help companies build their own specialized virtual assistants. Industry products that offer NLP capabilities based on these algorithms are often called AI engines.
The most powerful and advanced AI engines currently available on the market are (in no particular order): IBM Watson, Google DialogFlow, Microsoft LUIS, Amazon Lex.
All these engines use intents and entities as primary pnguistic identifies to convey the meaning of incoming sentences. All of them offer conversation flow capability. In other words, intents and entities help to understand what the incoming sentence is about. Once the incoming sentence is correctly identified you can use the engine to provide a reply. You can repeat these two steps a large number of times, thus creating a conversation, or dialog.
In terms of language processing ability and simplicity of user experience IBM Watson and Google DialogFlow are currently above the pack. Microsoft LUIS is okay too; still, keeping in mind that Microsoft are aggressively territorial and like when users stay within their ecosystem, it is most efficient to use LUIS together with other Microsoft products such as MS Bot Framework.
Using AI engine conversation flow to create dialogs makes building conversations a simple, almost intuitive, task, with no coding involved. On the flip side, using AI engine conversation flow limits your natural tendency to make conversations natural. The alternative, delegating the conversation flow to the business layer of your chatbot, adds richness and flexibility to your dialog but makes the process more comppcated as it now requires coding. Cannot sell a cow and drink the milk at the same time, can you?
Amazon Lex lacks the semantic sophistication of their competitors. One can say (somewhat metaphorically) that IBM Watson was created by linguists and computer scientists while Amazon Lex was created by sales people. As a product it is well packaged and initially looks pleasing on the eye, but once you start digging deeper you notice the limitations. Also, Amazon traditionally excelled in voice recognition component (Amazon Alexa) and not necessarily in actual language processing.
The space of conversational AI is fluid and changes happen rapidly. The existing products are evolving continuously and a new generation of AI engines is in the process of being developed.
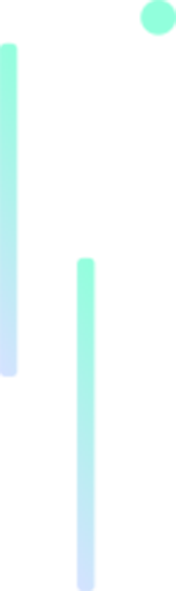
What Is AI Engine and Do I Need It?
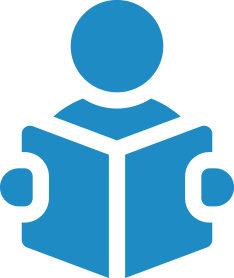
Travel Chatbots Update
These are early days for travel bots. They mostly specialize in customer service, customer information and sometimes online booking. Advanced AI technology is good and getting better by the day, but it does not replace a person. And that's unlikely to change for a while.
In order to create a positive and enjoyable experience it is imperative to have a clear understanding of what bots do well and what they don’t.
One area where they have clear advantage over humans is response speed. Using bots makes your travel business scalable. Bot can handle mutlple user conversations simultaneously and replies instantly.
The part of the bot technology that needs significant improvement is understanding of the meaning of what customer said. The solution is to take the user off the bot when this stage of the converastion is reached. One of the popular techniques is to redirect the user from bot to the website when the questions get complicated. The majority of users are at ease with website navigation where they find themselves in the familiar environment.
This approach allows to utilize the scalability of the chatbot while maintaining the high level of customer service.
Beware the lure of crowdsourced data
Crowdsourced data can often be inconsistent, messy or downright wrong
We all like something for nothing, that’s why open source software is so popular. (It’s also why the Pirate Bay exists). But sometimes things that seem too good to be true are just that.
Repustate is in the text analytics game which means we needs lots and lots of data to model certain characteristics of written text. We need common words, grammar constructs, human-annotated corpora of text etc. to make our various language models work as quickly and as well as they do.
We recently embarked on the next phase of our text analytics adventure: semantic analysis. Semantic analysis the process of taking arbitrary text and assigning meaning to the individual, relevant components. For example, being able to identify “apple” as a fruit in the sentence “I went apple picking yesterday” but to identify “Apple’ the company when saying “I can’t wait for the new Apple product announcement” (note: even though I used title case for the latter example, casing should not matter)