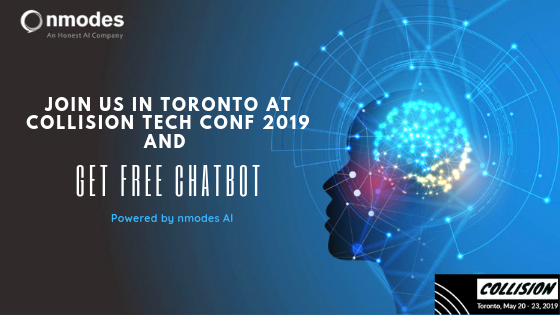
While Toronto is charged with hosting the Collision - "North America's fastest-growing tech conference" this year, nmodes is excited to make its first appearance among designated start-ups who have been selected to demo their products to conference visitors, potential investors, tech-enthusiasts and business executives.
nmodes, a year and a half in the market, offers a conversational product that uses AI to provide its customers with a scalable solution to execute 24/7/365 marketing acquisition and customer experience programs. While nmodes has already garnered its global presence with 40+ clients, North American market continues to be most enterprising for AI Chatbots and Voicebots. Collision Tech Event offers an exciting opportunity for nmodes team to take its networking game a notch higher and pitch it to businesses looking to catch-up with the AI space and be early adopters of hottest AI products available in the market.
How nmodes is different than other chatbots?
AI space is nothing new to the tech world as chatbots, virtual assistants and voice bots are finding their commercial contribution toward improving the customer experience of brands. nmodes continues to work closely with the businesses focusing on helping brands drive double digit growth in lead conversions and engagement rates.
Three key market differentiators for nmodes:
- 1. Interlacing marketing and customer experience
nmodes chatbots are custom built for the brands. nmodes solutions support full customer lifecycle from lead generation to marketing campaigns to scheduling demos, to gathering feedback and understanding engagement patterns of existing customers.
- 2. Lifetime AI training
nmodes solutions promise to work with progressive AI capabilities that are built to recognize old and new communication patterns and form a sensible response template that is malleable and fulfills the intent of desired conversation for the customers.
Nmodes solutions work on three principles while conversing with the customers.
A) Keep business context
nmodes solutions remember the customer’s history and their presence in the sales cycle and hence conversations are based upon the context of customer for the brand.
B) Data personalization
personalization of conversations focuses on collecting different data points from all internal and external data sources, helping brands deliver tailored and one-on-one predictive interactions.
C) Easy to use analytics
nmodes advanced dashboards uncover detailed analytics and insights on customer conversion rates, engagement rates and listen upon most common conversations to help brands better align their marketing communications and customer experience strategies.